At ConWX, we use multiple numerical weather predictions (NWP) data to calculate the optimal power forecasting for wind and solar. This is the industry standard, but our analysis also shows a very high value in choosing the right mix and having unique NWP input for power forecasting.
The industry typically uses machine learning for optimizing each power plant before aggregating to the portfolio level with statistical mixing. In China, AI for training is more widely used than in Europe; however, the performance is at the same level for both ML and AI training.
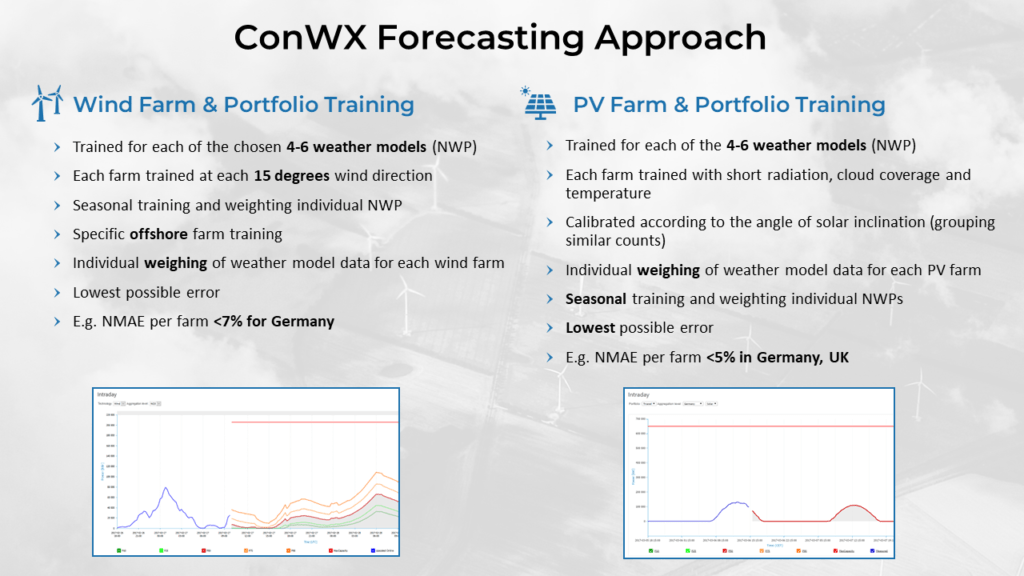
Weather model data mix
The reason for mixing different power forecasts from different NWPs or different forecasting vendors is obviously to reduce the average errors in day-to-day forecasts and not to rely solely on one source. As the chaotic behaviour of the atmosphere introduces high uncertainty into all NWPs, even the best ones in benchmarking. The disadvantage of having too many different power forecasts is also that you will not always accurately predict peaks and lows.
We all know that there is one dominant NWP, the ECMWF model. This is the model that performs the best on average, but it cannot do the work alone. For instance, over a year, we observe the following day ahead NME (normalized mean errors) for a 250 MW wind portfolio
Volatile energy market
Since 2022, with the rise in power prices and an increased number of negative hours both day and night, the importance of assessing risks in power forecast development has grown significantly. Given that ECMWF is the most widely used Numerical Weather Prediction (NWP) model. Its failure can lead to costly consequences as the market heavily relies on it.
A recent analysis by our data scientists Marcus Garsdal and Dimitris Voukatas underscores the economic impact of selecting the right mix and calculating potential earnings for a small power portfolio consisting of wind and solar, totalling around 150 MW. Please note that all data presented are based on our day-ahead forecasts and do not account for any intraday trading activities.
The balancing costs within the energy market depend on your position in relation to the balancing market, as explained in the figure below for the German market.

The balancing market in Germany, covering the period from January 1, 2021, to December 31, 2022, vividly illustrates the volatility observed during this two-year timeframe. Notably, the volatility surged during the winter of 2021/2022, with a sharp acceleration starting at the end of February, coinciding with the invasion of Ukraine in February 2022. This heightened volatility both increases the market’s risks and presents opportunities.
ConWX vs. ECMWF weather model forecast
Examining the cumulative income and earnings for this portfolio and comparing the power model based on our best in-house Numerical Weather Prediction (NWP) and ECMWF over the two-year period clearly highlights the value of having a unique NWP exclusive to our operation.
The cost and earnings graphs for both models, ConWX (green and purple) and ECMWF (pink and yellow) follow a nearly identical trajectory initially. However, as volatility increases and both costs and incomes fluctuate, the ConWX-based Day-Ahead Hourly (DAH) model ultimately outperforms, resulting in accumulated earnings of 0.6 million Euros better.

The analysis also reveals some costly days, particularly around February 18, 2022, where the portfolio incurred a loss of 0.25 million Euros over a few days.
With more renewables on the grid, we anticipate even greater volatility in the market, making it more crucial than ever to choose the right model. In our R&D team, we are currently focused on uncertainty flagging and leveraging Graph Neural Networks to optimize short-term forecasts.